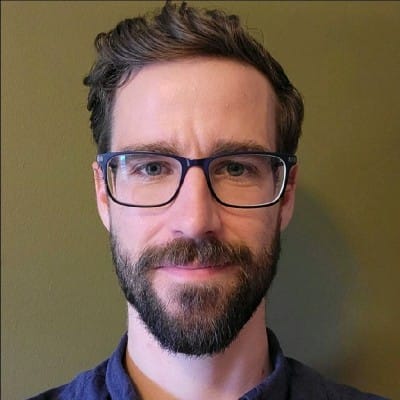
Craig Sinnamon
Power TakeOff | Director of Analytics and Data Management
Speaker
Track B: Emerging Technologies and Renewable Energy
Session B2: Artificial Intelligence, Big Data, and Tracking
May 2, 2024 | 10:00 am - 10:30 am
Unlocking Electrification with Hourly Emissions Data
International agencies are working to create a sense of urgency toward climate action. Specific actions such as replacing fossil-fuel-fired heating equipment with heat pumps, electrifying transportation, and energy storage are recommended. These broad actions will be evaluated and implemented at individual facilities. The carbon impact of buildings must include the embodied carbon of the steel, wood, glass, and concrete used in construction.
But you can’t manage what you measure incorrectly.
Currently the dominant method for measuring carbon emissions is to multiply energy consumption for each fuel by an emissions factor, using the average contributors to electricity generation. Recent standards have recommended utilizing long-run marginal contributions to electrical generation (“consequential analysis”). This study addresses the question: How much difference would this change make?
We performed a comparative analysis using a sample of approximately 30,000 buildings. We calculated emissions data from two distinct methods: the eGRID (2021 annual average factors) and the Cambium (8-year average, 2023-2030) hourly long-run marginal emissions factors (as recommended in the new standards).
This comparison tests the hypothesis that using average impacts undercuts global policy goals such as electrification.
We found a significant divergence between the two datasets that requires urgent action to ensure that the M&V choices we make aren’t jeopardizing investment in energy efficiency projects that actually combat climate change. The conclusion found is that all studied regions show that we will encourage electrification more if we use hourly emissions data rather than annual averages.
Speaker Bio
Craig Sinnamon has a background in mathematics and has worked to advance methodology and basic assumptions around weather data and how to model the impacts of energy efficiency programs using machine learning and big data analysis. His primary research focuses have been around adapting for grid level impacts including non-routine events, improving modeling methodologies to utilize high granularity advanced metering infrastructure data, updating weather sources used for savings claims and grid planning, and decarbonization data sources. He is currently employed as the Director of Analytics and Data Management at Power TakeOff, a utility implementation contractor.